In the realm of technology, two terms often intermingle and are sometimes used interchangeably, leading to confusion among many: Machine Learning (ML) and Artificial Intelligence (AI). While they are closely related and share some similarities, they are distinct concepts with different goals and applications. In this article, we will delve into the world of ML and AI, exploring their differences, similarities, and the roles they play in shaping our future.
Table of Contents:
We invite you to read: “HOW AI AND MACHINE LEARNING ARE CHANGING THE FACE OF BUSINESS”
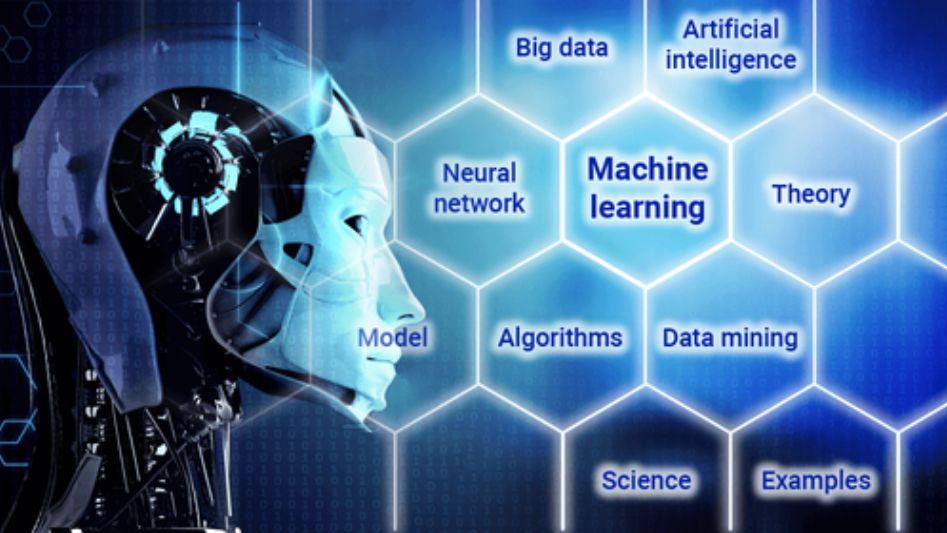
Understanding Artificial Intelligence (AI)
Artificial Intelligence, often referred to as AI, is a broad field of computer science that aims to create machines or systems capable of performing tasks that typically require human intelligence. These tasks include reasoning, problem-solving, learning from experience, understanding natural language, recognizing patterns, and making decisions. AI seeks to replicate human-like intelligence in machines, allowing them to adapt and perform a wide range of tasks without being explicitly programmed for each one.
The Scope of AI
AI can be categorized into two main types: Narrow or Weak AI and General or Strong AI.
- Narrow AI (Weak AI): Narrow AI systems are designed for specific tasks. They excel in one particular area, such as image recognition, natural language processing, or playing chess. These systems do not possess general intelligence and cannot perform tasks outside their designed scope.
- General AI (Strong AI): General AI, on the other hand, is the ultimate goal of AI research. It refers to machines that possess human-level intelligence and can perform any intellectual task that a human can do. This level of AI is still largely theoretical and has not been achieved as of my knowledge cutoff date in January 2022.
Exploring Machine Learning (ML)
Machine Learning is a subset of Artificial Intelligence that focuses on the development of algorithms and statistical models that enable computers to learn from and make predictions or decisions based on data. In essence, ML enables machines to improve their performance on a specific task by learning from experience, rather than relying on explicit programming.
Key Concepts in Machine Learning
To understand the workings of ML, it’s essential to grasp some fundamental concepts:
- Training Data: ML models require large datasets for learning. These datasets are used to train the model, allowing it to recognize patterns and make predictions.
- Algorithms: ML algorithms are mathematical models that process the training data and adjust their parameters to optimize their performance on the task at hand.
- Feature Engineering: Feature engineering involves selecting and transforming relevant data attributes (features) to improve the model’s ability to make accurate predictions.
- Supervised, Unsupervised, and Reinforcement Learning: ML can be categorized into different types, depending on the learning approach. Supervised learning involves training models on labeled data, unsupervised learning deals with unlabeled data, and reinforcement learning focuses on learning through interaction with an environment.
We invite you to read: “THE ROLE OF AI IN MARKETING: USING MACHINE LEARNING TO DRIVE SALES”
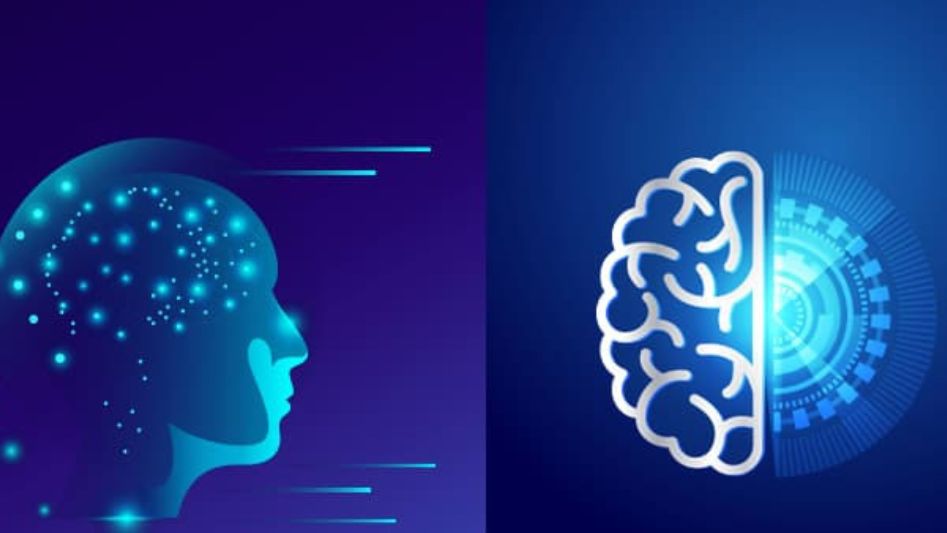
Key Differences Between AI and ML
Now that we have a foundational understanding of AI and ML, let’s highlight the key differences between the two:
1. Scope and Purpose
- AI: AI aims to create machines with human-like intelligence, capable of performing a wide range of tasks across various domains.
- ML: ML focuses on the development of algorithms that enable machines to learn and improve their performance on specific tasks through data analysis.
2. Learning and Adaptation
- AI: AI systems are typically rule-based and rely on predefined instructions and programming.
- ML: ML systems learn and adapt based on data, making them more flexible and capable of handling complex, data-driven tasks.
3. Examples
- AI: Virtual assistants like Siri or Alexa, self-driving cars, and chatbots are examples of AI applications.
- ML: Recommender systems (used by Netflix and Amazon), spam email filters, and image recognition algorithms are examples of ML applications.
4. Level of Intelligence
- AI: AI systems strive to achieve human-level intelligence and reasoning, which is a challenging goal that has not yet been fully realized.
- ML: ML systems focus on specific tasks and do not possess general intelligence.
The Synergy of AI and ML
While AI and ML have distinct differences, they are not mutually exclusive. In fact, ML plays a pivotal role within the field of AI. Machine learning techniques are often used to achieve AI’s goal of creating intelligent systems. For example, natural language processing (NLP) models like GPT-3 use ML algorithms to understand and generate human-like text, contributing to AI applications in language understanding and generation.
We invite you to read: “THE FUTURE OF AI IN BUSINESS: HOW MACHINE LEARNING IS CHANGING THE GAME”
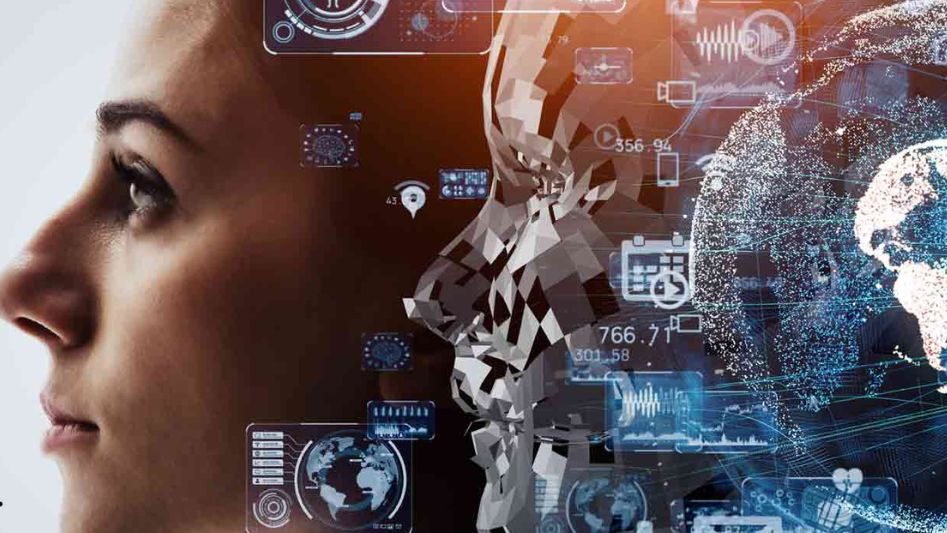
Conclusion
In summary, Machine Learning and Artificial Intelligence are intimately connected, with ML being a subset of AI. AI encompasses a broader vision of creating intelligent machines, while ML focuses on the development of algorithms that enable machines to learn and make predictions from data. Understanding the distinctions between these two fields is crucial for grasping their respective applications and potential impacts on society. As technology continues to advance, the collaboration between AI and ML will likely shape the future of innovation and automation in numerous industries.
FAQs
How do AI and ML differ?
AI has a broader goal of replicating human-like intelligence, while ML specializes in creating algorithms that enable machines to learn from data. AI encompasses various technologies, including ML.
Can you provide examples of AI and ML applications?
AI applications include virtual assistants like Siri and self-driving cars, while ML applications include recommendation systems (e.g., Netflix recommendations) and image recognition software.
Are AI and ML related?
Yes, they are related. ML is a key component of AI, as it provides the learning and prediction capabilities necessary to achieve AI’s goal of creating intelligent systems.
You May Also Like
- AI IN FINANCE: HOW MACHINE LEARNING IS CHANGING THE BANKING INDUSTRY
- PYTHON FOR MACHINE LEARNING: BUILDING INTELLIGENT SYSTEMS FROM SCRATCH
- MACHINE LEARNING IN CYBERSECURITY: DEFENDING AGAINST EVOLVING THREATS
- MACHINE LEARNING VS. ARTIFICIAL INTELLIGENCE: UNDERSTANDING THE DIFFERENCE
- AI AND MACHINE LEARNING: SOLVING REAL-WORLD PROBLEMS
Recent Comments