In today’s digital age, the rapid advancement of technology has revolutionized the way we live, work, and communicate. However, along with these advancements come new and complex challenges, particularly in the realm of cybersecurity. As cyber threats continue to evolve and become more sophisticated, organizations and individuals must stay one step ahead to protect sensitive information and secure their digital assets.
Table of Contents:
We invite you to read: “MACHINE LEARNING VS. ARTIFICIAL INTELLIGENCE: UNDERSTANDING THE DIFFERENCE”
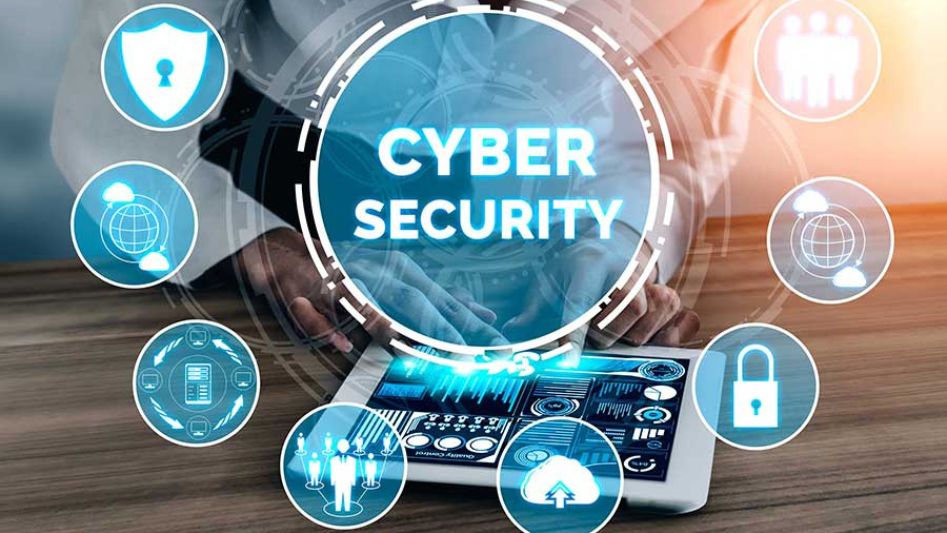
The Role of Machine Learning in Cybersecurity
Machine learning, in the context of cybersecurity, involves the use of algorithms and statistical models to enable computers to learn from data and make informed decisions. Traditional rule-based systems are often insufficient to handle the dynamic and evolving nature of cyber threats. Machine learning algorithms, on the other hand, can analyze vast amounts of data, identify patterns, and detect anomalies that may indicate a potential security breach.
Enhancing Threat Detection
One of the primary applications of machine learning in cybersecurity is enhancing threat detection capabilities. Traditional signature-based detection methods rely on known patterns and signatures to identify threats. However, these methods often fail to detect new and emerging threats. Machine learning algorithms can overcome this limitation by continuously learning from new data and adapting their detection mechanisms accordingly.
By training machine learning models on a wide range of data, including historical attack data, network traffic, and system logs, cybersecurity systems can identify suspicious activities that deviate from normal behavior. These models can learn to recognize patterns associated with different types of attacks, enabling early detection and timely response.
Predictive Analytics for Proactive Defense
Machine learning can also enable predictive analytics, empowering organizations to adopt a proactive approach to cybersecurity. By analyzing historical data and identifying trends, machine learning models can predict potential vulnerabilities and future attack vectors. This foresight allows organizations to implement preemptive measures and strengthen their defenses before an attack occurs.
Predictive analytics can also assist in identifying zero-day vulnerabilities, which are previously unknown vulnerabilities that hackers can exploit. Machine learning models can analyze system behavior and identify anomalies that may indicate the presence of zero-day exploits, providing an opportunity for proactive patching and mitigation.
Automated Incident Response
In the face of a cyber attack, swift and effective incident response is crucial to minimize damage and restore normal operations. Machine learning can automate the incident response process by analyzing incoming data, identifying the severity of the threat, and initiating appropriate actions.
Through the integration of machine learning algorithms with security orchestration and automation platforms, organizations can automate routine response tasks, such as isolating compromised systems, blocking suspicious network traffic, and generating incident reports. This automation not only speeds up the response time but also reduces the risk of human error, ensuring a more efficient and effective incident management process.
We invite you to read: “HOW AI AND MACHINE LEARNING ARE CHANGING THE FACE OF BUSINESS”
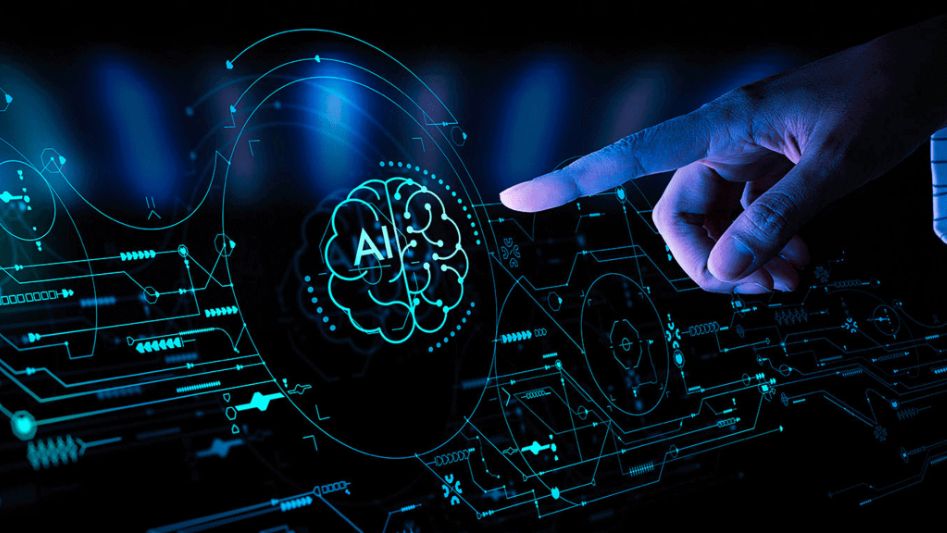
Challenges and Limitations
While machine learning offers significant benefits in the realm of cybersecurity, it also faces several challenges and limitations that need to be addressed for optimal implementation.
Adversarial Attacks
Adversarial attacks refer to techniques employed by malicious actors to manipulate machine learning models and evade detection. By exploiting vulnerabilities in the learning process, adversaries can craft inputs specifically designed to fool the algorithms and bypass security measures.
To defend against adversarial attacks, cybersecurity professionals must continuously update and refine machine learning models, improving their robustness and resistance to manipulation. Adversarial training, where models are exposed to deliberately crafted adversarial samples during training, can help improve the model’s ability to detect and mitigate such attacks.
Data Quality and Bias
Machine learning models heavily rely on the quality and diversity of the training data. If the training data is biased or incomplete, the model’s performance may be compromised, leading to false positives or false negatives in threat detection.
To mitigate this challenge, organizations must ensure they have access to high-quality and representative data sets for training their machine learning models. Furthermore, ongoing monitoring and validation of the model’s performance against new and diverse data sources are essential to identify and address any biases that may arise.
Privacy and Ethical Considerations
Machine learning algorithms often require access to sensitive data to perform effective threat detection. However, this raises concerns regarding privacy and ethical considerations. Organizations must strike a balance between leveraging machine learning for enhanced cybersecurity and preserving the privacy and confidentiality of user data.
Implementing privacy-preserving techniques, such as data anonymization and differential privacy, can help address these concerns. Additionally, organizations should adhere to legal and ethical guidelines and ensure transparent communication with users regarding the collection and usage of their data.
We invite you to read: “THE ROLE OF AI IN MARKETING: USING MACHINE LEARNING TO DRIVE SALES”
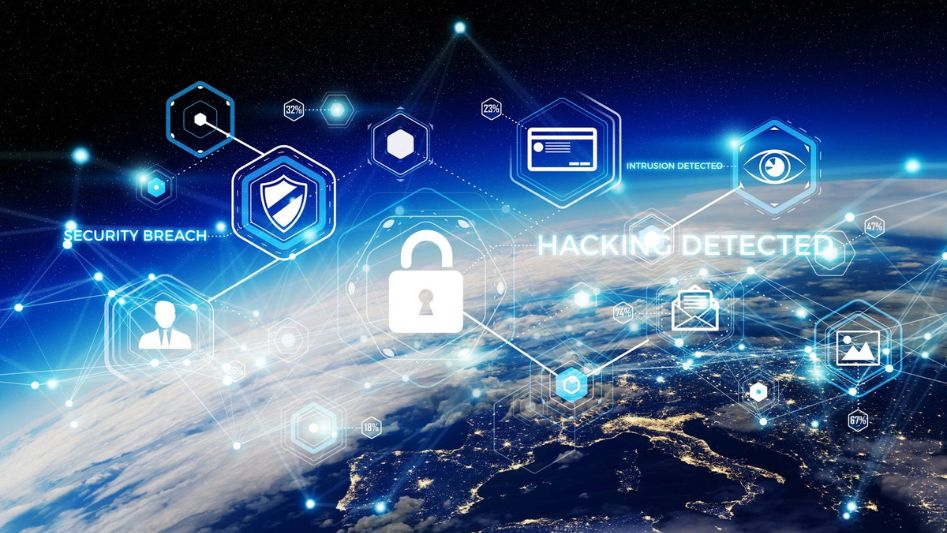
Conclusion
Machine learning has become an indispensable tool in the fight against evolving cyber threats. By leveraging the power of algorithms and data analysis, cybersecurity professionals can enhance threat detection, adopt proactive defense strategies, and automate incident response. However, challenges such as adversarial attacks, data quality, and privacy considerations must be addressed to ensure the effective and ethical implementation of machine learning in cybersecurity.
As technology continues to advance and threats become increasingly sophisticated, organizations must embrace innovative solutions like machine learning to defend against evolving cyber threats effectively. By staying vigilant, investing in robust cybersecurity measures, and leveraging the power of machine learning, we can create a safer digital landscape for all.
FAQs
What is machine learning in the context of cybersecurity?
Machine learning in cybersecurity refers to the use of algorithms and statistical models to enable computers to learn from data and make informed decisions in the realm of digital security. It involves analyzing large volumes of data to detect patterns, anomalies, and potential threats.
How does machine learning enhance threat detection in cybersecurity?
Machine learning enhances threat detection in cybersecurity by continuously learning from new data and adapting detection mechanisms accordingly. It can analyze vast amounts of data, identify patterns, and detect anomalies that may indicate a potential security breach, including both known and emerging threats.
Can machine learning predict future cyber attacks?
Yes, machine learning can enable predictive analytics in cybersecurity, allowing organizations to predict potential vulnerabilities and future attack vectors. By analyzing historical data and identifying trends, machine learning models can provide insights that empower organizations to implement preemptive measures and strengthen their defenses.
How can machine learning automate incident response?
Machine learning can automate incident response by analyzing incoming data, identifying the severity of threats, and initiating appropriate actions. By integrating machine learning algorithms with security orchestration and automation platforms, organizations can automate routine response tasks, minimizing response time and reducing the risk of human error.
You May Also Like
- THE FUTURE OF AI IN BUSINESS: HOW MACHINE LEARNING IS CHANGING THE GAME
- HOW TO APPLY MACHINE LEARNING TO BUSINESS PROBLEMS. TOP #3 KEYS ELEMENTS.
- AI AND MACHINE LEARNING: SOLVING REAL-WORLD PROBLEMS
- THE FUTURE OF ARTIFICIAL INTELLIGENCE: A LOOK AT ADVANCEMENTS IN MACHINE LEARNING
- THE POWER OF NATURAL LANGUAGE PROCESSING: MAKING MACHINES UNDERSTAND US
Recent Comments