Natural Language Processing (NLP) is a subfield of artificial intelligence (AI) that focuses on enabling machines to understand, interpret, and generate human language. Over the years, NLP has witnessed remarkable advancements, transforming the way we interact with technology and revolutionizing various industries. This article explores the evolutionary journey of NLP in AI, from its inception to its current state and beyond.
Table of Contents:
We invite you to read: “THE EVOLUTION OF SMARTPHONES: FROM COMMUNICATION DEVICES TO POCKET COMPUTERS”
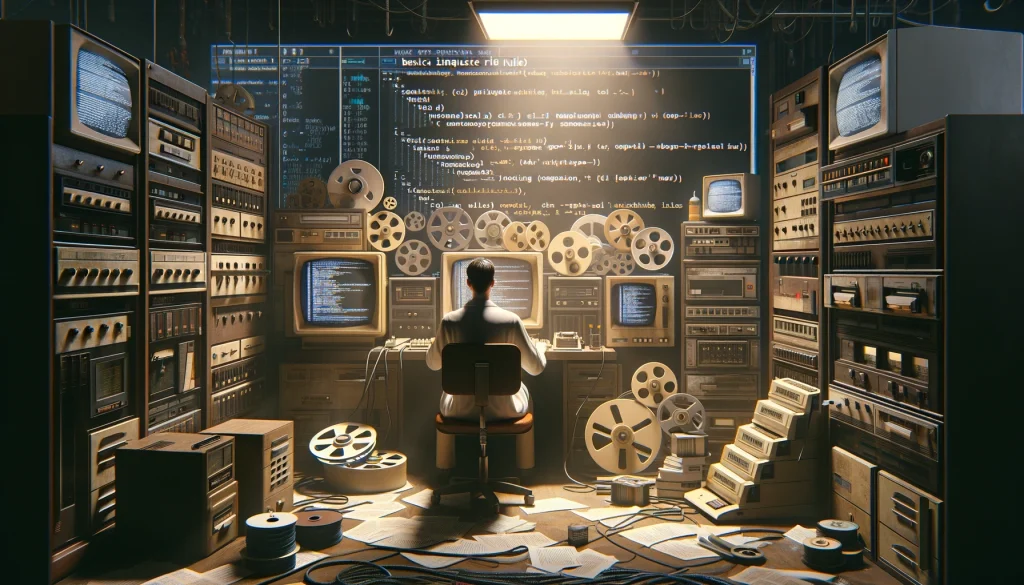
The Birth of NLP
Early Beginnings
The roots of NLP can be traced back to the mid-20th century, with early efforts aimed at developing machines that could understand and respond to human language. Researchers like Alan Turing and Warren Weaver laid the foundation for this field by envisioning the possibility of machines that could simulate human thought processes.
Rule-Based Systems
In the 1950s and 1960s, NLP predominantly relied on rule-based systems. These systems utilized grammatical and syntactical rules to process and generate text. While they showed promise, they were limited in their ability to handle the complexity and nuances of natural language.
The Statistical Revolution
The Rise of Machine Learning
The 1990s marked a significant turning point in NLP with the advent of machine learning techniques. Researchers began to use statistical models to analyze and understand language. This shift allowed NLP systems to learn from vast amounts of data, making them more adaptable and capable of handling real-world language variations.
Hidden Markov Models and Part-of-Speech Tagging
During this period, Hidden Markov Models (HMMs) became popular for tasks like part-of-speech tagging and speech recognition. These statistical models allowed for more accurate and context-aware language processing.
The Deep Learning Era
Introduction of Neural Networks
The real breakthrough in NLP came with the introduction of neural networks, especially deep learning models. Recurrent Neural Networks (RNNs) and Convolutional Neural Networks (CNNs) began to gain prominence in the 2010s. These models, along with advances in hardware and the availability of massive datasets, led to substantial improvements in natural language understanding.
Word Embeddings
Word embeddings, such as Word2Vec and GloVe, emerged as key technologies during this era. These techniques transformed the way machines understood words by representing them as dense vectors, capturing semantic relationships between words.
Transformer Architecture
The pinnacle of NLP’s evolution arrived with the introduction of the Transformer architecture in 2017. The Transformer model, most famously represented by BERT (Bidirectional Encoder Representations from Transformers), revolutionized NLP by enabling deep learning models to capture context from both directions within a sentence, leading to unprecedented language understanding and generation capabilities.
We invite you to read: “THE EVOLUTION OF MOBILE TECHNOLOGY: FROM 1G TO 5G”
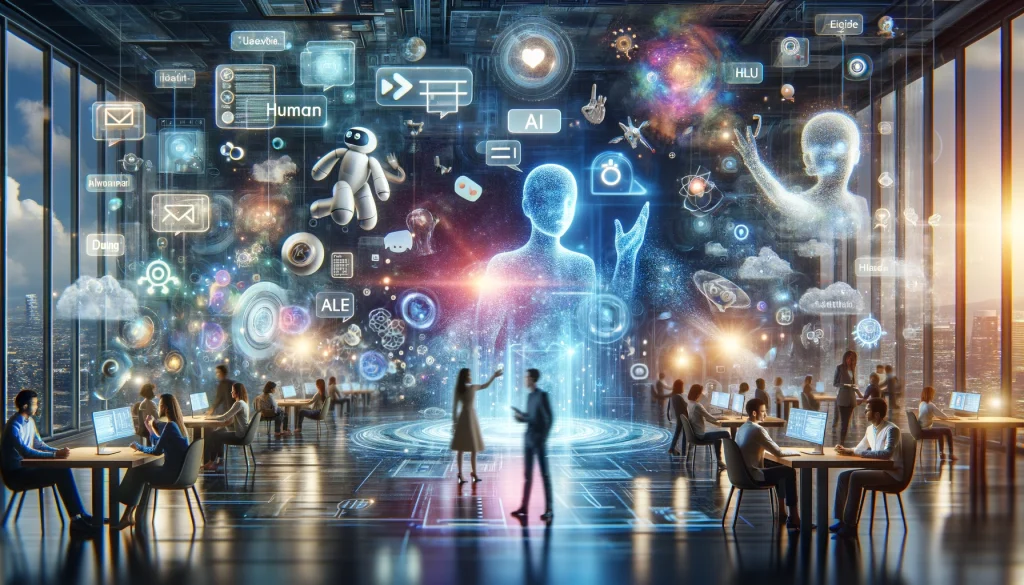
Current State and Applications
Pre-trained Language Models
Today, pre-trained language models like GPT-3, T5, and XLNet have become powerhouses of NLP. These models, trained on vast corpora of text, can perform a wide range of tasks, including text generation, translation, question answering, and sentiment analysis with remarkable accuracy.
Chatbots and Virtual Assistants
NLP has paved the way for the development of intelligent chatbots and virtual assistants like Siri, Alexa, and Google Assistant. These AI-powered entities can engage in natural conversations with users, making them an integral part of our daily lives.
Sentiment Analysis and Customer Insights
Businesses harness NLP to gain valuable insights from customer reviews and social media sentiments. This technology helps companies understand customer preferences, track brand reputation, and improve products and services.
Future Prospects
Multimodal Understanding
The future of NLP in AI is expected to include multimodal understanding. This means combining text with other forms of data like images and videos to create AI systems that can comprehend and generate content across various modalities.
Ethics and Bias Mitigation
As NLP models become more prevalent, addressing ethical concerns and mitigating biases in AI becomes crucial. Ongoing research is focusing on developing fairness-aware NLP models and ensuring equitable language processing.
Human-AI Collaboration
NLP advancements are expected to lead to better human-AI collaboration, with AI systems assisting humans in more complex language-related tasks, such as content generation, translation, and data analysis.
We invite you to read: “THE EVOLUTION OF CLOUD COMPUTING: WHAT’S NEXT FOR THE CLOUD?”
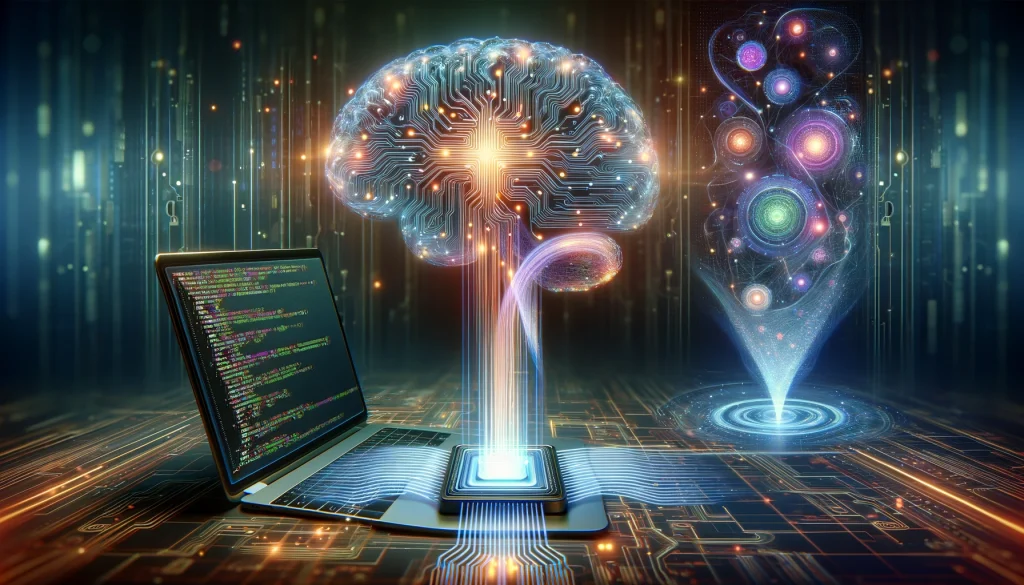
Conclusion
The evolution of Natural Language Processing in AI has been a remarkable journey, transforming the way we interact with machines and enhancing our daily lives. From rule-based systems to deep learning models, NLP has come a long way, and its future promises even more exciting possibilities. As NLP continues to advance, it will play an increasingly significant role in shaping the future of AI and human-computer interaction.
FAQs
How has NLP evolved over time?
NLP has evolved from rule-based systems to statistical models and, more recently, to deep learning techniques, resulting in significant improvements in language understanding and generation.
What are some current applications of NLP?
NLP is used in chatbots, virtual assistants, sentiment analysis, translation, and customer insights, among other applications.
What does the future hold for NLP in AI?
The future of NLP includes multimodal understanding, addressing ethics and biases, and fostering better human-AI collaboration.
How can NLP benefit businesses and industries?
NLP can provide valuable insights, enhance customer engagement, automate tasks, and improve decision-making in various sectors.
You May Also Like
- THE SILENT REVOLUTION: HOW IOT DEVICES ARE QUIETLY CHANGING OUR DAILY LIVES
- BIG DATA IN MARKETING: A GOLDMINE OF CUSTOMER INSIGHTS
- CLOUD COMPUTING: EMPOWERING BUSINESSES AND INDIVIDUALS
- BLOCKCHAIN BEYOND CRYPTOCURRENCY: EXPLORING THE POTENTIAL OF DISTRIBUTED LEDGER TECHNOLOGY
- THE ROLE OF AI IN SHAPING SUSTAINABLE FUTURES
Recent Comments