The term deep learning has been around for a while now, but what exactly is it? And why is it such a hot topic right now? Deep learning is a machine learning technique that extracts valuable information from data.
Likewise, deep learning is a type of artificial intelligence that has been gaining attention for the last few years. The technology applies to several areas, including computer vision, speech recognition, and robotics.
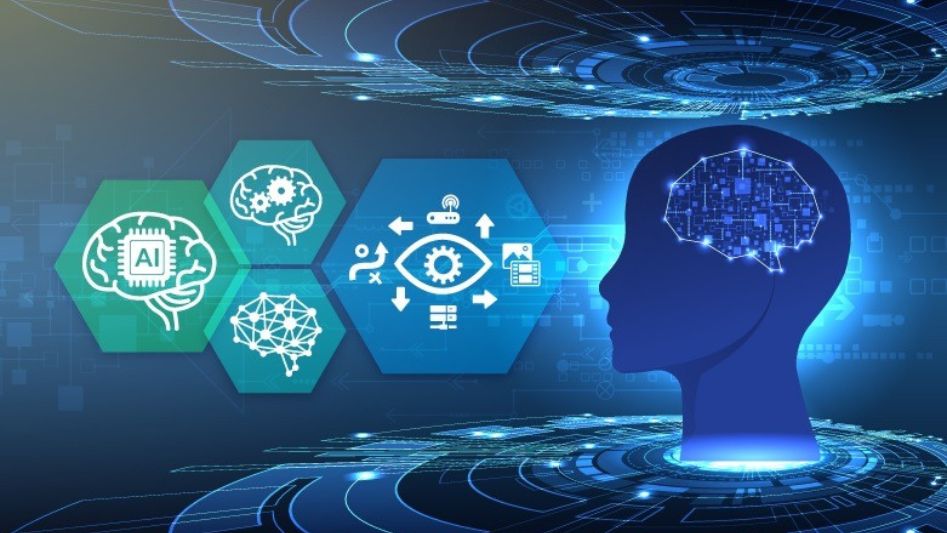
Today, we will discuss deep learning. The branch of machine learning is a subset of artificial intelligence (AI). Deep learning is a subset of AI.
TABLE OF CONTENTS:
- Deep Learning
- Second Name of Deep Learning
- Benefits of Deep Learning
- Limitations of Deep Learning
- Application of Deep Learning
- Future of Deep Learning
- Conclusion
- FAQ
Deep Learning
Deep Learning is a branch of Machine Learning, Artificial Intelligence, and Neurocomputing. It is also known as Deep Neural Network (DNN) or Deep Artificial Neural Network (DAANN). This new technology is for various fields, such as Computer Vision, Speech Recognition, Natural Language Processing, etc.
It’s true that deep learning is the most recent development in artificial intelligence, which is an area of computer science that focuses on the design and training of algorithms that can learn from data.
Although, Artificial intelligence is to automate tasks and make computers capable of performing tasks that humans previously did. It has many applications in fields such as speech recognition, machine translation, image processing, and natural language processing.
In this article, you learn about the basics of Deep Learning and its applications.
Second Name of Deep Learning
Deep learning is also known as artificial neural networks. In simple terms, it’s the ability to take all the information available to a computer and put it through an algorithm to come up with the best answer.
Benefits of Deep Learning
- One of the reasons why deep learning is so powerful is because it learns from experience.
- Like a human child, it develops knowledge and understanding through trial and error.
- Once it gets something right, it keeps doing it until it doesn’t need to keep doing it anymore.
- In the same way that a human child’s brain can’t understand the concept of “negative numbers” until it’s old enough to learn math, the deep learning algorithms in AI technology are only able to learn what they need to know for their tasks, such as recognizing the difference between an animal and a car.
Limitations of Deep Learning
- To answer it, deep learning needs improvements.
- It’s only one way to tackle machine learning problems.
- An excellent tool for learning how to implement machine learning, but it has limitations.
For example, deep learning models need to improve at handling images, videos, or audio files with too many data points or needing more training.
Application of Deep Learning
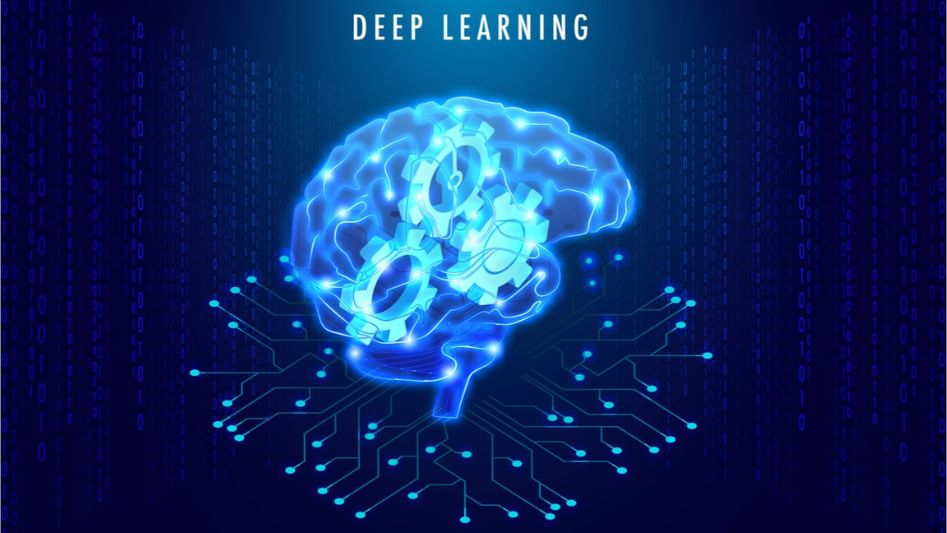
When a human face a problem, they look at all available information and then decide based on it. A deep learning model uses the same approach, except instead of making decisions, it predicts what will happen in the future.
We can say deep learning is a relatively new type of artificial intelligence (AI) that is being applied to a wide range of problems.
For example, the technology predicts weather patterns, recognizes images, detects fraud, and classifies diseases.
Let’s discuss some of these in detail:
- Self-Driving Cars
First of all, the self-driving car is an excellent example of deep learning. It’s a unique technology to help self-driving cars learn how to navigate roads. It uses artificial intelligence and big data.
- HealthCare
Secondly, deep learning is already used to help doctors diagnose diseases, predict hospital readmission rates, and improve medical care.
By using deep learning, researchers are developing systems that can make diagnoses based on the shape of data instead of just looking at it. For example, these systems can tell if a patient is suffering from diabetes or heart failure without having to run tests.
- Language Processing
We’re in the middle of a vast technological revolution in machine learning, and the applications of this technology will only increase.
However, it is also true in other areas of data science, such as Natural Language Processing (NLP). If you need an idea of NLP. It’s an area of study that has been around for over a century. NLP is the science of understanding human language and using it to create intelligent software systems that can process, analyze, and understand natural language text.
- Pixel Restoration
Deep learning has emerged as a powerful new tool for image restoration. These techniques are especially effective at removing noise and restoring details. They also allow for faster image processing and are particularly adept at removing blur and blurring.
In addition, these techniques are used to remove blur from photos, restore details from images that the effects of noise have degraded, and even detect objects in images.
5. Deep Dreaming
Lastly, Deep Dreaming is a visualization of images that uses neural networks to interpret the image. These networks are trained on large datasets of images, and the goal is to train them.
For example, they associate the image they see with the object or scene with it.
Future of Deep Learning
After all of its pros and cons, it is valuable to say that deep learning is the new normal. Moreover, it will be widely applicable to commercial and private sectors, as the two main types include: supervised and unsupervised.
- Supervised deep learning uses labeled data (data tagged with its class) to learn.
- In contrast, unsupervised deep learning uses unlabeled data to learn.
Conclusion
In conclusion, there are many applications of deep learning. For example, it is used in image recognition, language translation, and speech recognition. In addition, deep learning also plays an essential role in computer vision and robotics.
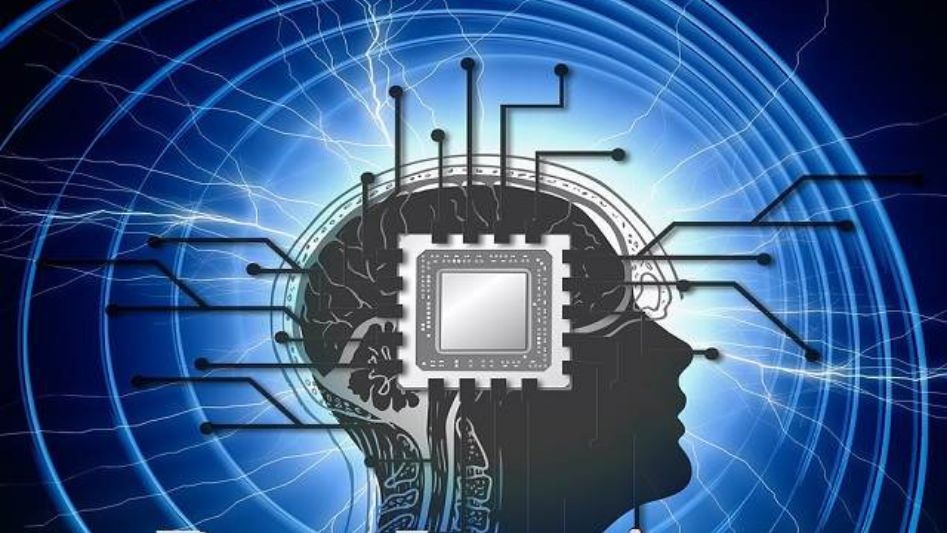
FAQ
What is Deep Learning, and what is it used for?
Deep learning is a subfield of machine learning that uses artificial neural networks. It is for computer vision, speech recognition, and natural language processing.
What are the two phases of deep learning?
Deep learning consists of two parts: training and testing. First, the training phase involves creating a model and training it on a data set. Secondly, the testing phase involves using the model to analyze new data.
What is the difference between Deep Learning and Machine Learning?
There are three significant differences between deep learning and machine learning: Deep learning is usually applied to datasets with a lot of variation in the features. Machine learning can be used to classify and predict categorical data. Deep learning is often applied to tasks where the data is unstructured.
What is Artificial Neural Network?
An artificial neural network (ANN) is a computational model inspired by the biological neural networks in our brains. ANNs are usually composed of layers and groups of nodes connected by weighted connections.
You May Also Like
5 AI Apps That Will Change In Daily Life
6 IoT Projects For Beginners, Easy But Effective Ones
IoT Solutions For Climate Change – Clean, Green Environment
5 Basic Data Science Projects in Python for Beginners
Building a Security System With ESP32 Camera [Parts & Guide]
Helpful Links:
Recent Comments